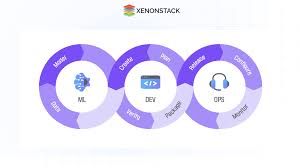
As AI agents become more autonomous and capable, organizations are increasingly deploying them across diverse workflows—from customer support and data analysis to IT automation and content generation. But as with any growing digital workforce, scaling AI agents comes with operational challenges.
Enter AgentOps—a new and emerging discipline focused on the management, monitoring, and optimization of autonomous AI agents at scale.
What Is AgentOps?
AgentOps (short for Agent Operations) is the framework, tools, and practices used to deploy, manage, monitor, and refine AI agents running in dynamic environments. Similar to DevOps in software engineering, AgentOps ensures that AI agents perform reliably, remain aligned with business goals, and adapt to changing conditions.
Why AgentOps Matters
1. Scalability
As businesses adopt dozens or even hundreds of AI agents, managing them manually becomes unsustainable. AgentOps enables centralized control and orchestration.
2. Accountability
Autonomous agents must remain aligned with organizational rules and ethical standards. AgentOps includes real-time monitoring and feedback loops to ensure agents don’t deviate from intended behavior.
3. Reliability
Like human employees, AI agents can encounter errors, get stuck, or behave unpredictably. AgentOps incorporates fail-safes, error handling, and recovery mechanisms to maintain performance.
4. Continuous Improvement
Through analytics and logging, businesses can track agent performance, analyze bottlenecks, and improve workflows using data-driven insights.
Key Components of an AgentOps Stack
- Agent Orchestration Platforms (e.g., AutoGen, CrewAI, LangChain): Coordinate task execution across multiple agents.
- Monitoring Dashboards: Provide visibility into agent activity, status, performance metrics, and error rates.
- Version Control: Manage updates and track changes to agent behavior, prompts, and configurations.
- Human-in-the-Loop (HITL) Systems: Enable manual oversight or intervention when needed.
- Logging & Analytics: Capture real-time data to audit decisions, refine processes, and ensure compliance.
Challenges in AgentOps
- Security Risks: Ensuring agents don’t access or misuse sensitive data.
- Ethical Alignment: Preventing unintended consequences from autonomous decision-making.
- Inter-Agent Conflicts: Managing coordination and avoiding duplicated or conflicting tasks.
- Latency & Performance: Optimizing communication and response times, especially in mission-critical use cases.
Use Cases Benefiting from AgentOps
- Customer Support: Monitor performance of AI-driven chatbots and escalate issues when needed.
- Marketing Automation: Oversee content generation agents across platforms with consistency.
- IT Management: Ensure automated diagnostic and remediation agents work efficiently and safely.
- E-commerce: Manage agents that handle product recommendations, order processing, and fraud detection.
The Future of AgentOps
At Greelogix, we see AgentOps as the backbone of enterprise-scale AI agent adoption. As autonomous agents continue to evolve, businesses will require mature systems to keep them efficient, secure, and aligned with human goals.
Whether you’re deploying your first AI agent or scaling a fleet across departments, AgentOps is key to achieving reliable, responsible, and scalable AI operations.
Ready to Scale AI Agents for Your Business?
Let Greelogix help you design and implement a robust AgentOps framework that empowers your autonomous agents while ensuring trust and control.